from maxrf4u import get_patterns, plot_patterns, all_elements
The peak pattern puzzle
We now arrive at the central problem of MA-XRF analysis. Given a spectrum with some peaks, and given the theoretical emission peak patterns for different chemical elements, which chemical elements are present in the sample? This is what I call the peak pattern puzzle.
It is important to note that not all peaks are always due to the actual emission of chemical elements present in the scanned object. Other peaks are generated by the instrument itself. And small peaks can also just be noise. Instrument peaks are common to all spectra. For example, the large peak observed in all spectra near zero energy is a result of the instrument detector physics. Other peaks above 18 keV are due emission and subsequent scattering of the rhodium anode present in the x-ray tube.
In order to plot an overview of emission patterns import plot_patterns()
. To simplify our analysis let’s exclude the light elements and rare elements that we will not find in drawings.
Code
# elements of interest
# adapt selection by (un)commenting
'''
all_elements = ['#H', '#He', '#Li', '#Be', '#B', '#C', 'N', 'O', 'F', 'Ne', 'Na', 'Mg',
'Al', 'Si', 'P', 'S', 'Cl', '#Ar', 'K', 'Ca', '#Sc', 'Ti', 'V', 'Cr',
'Mn', 'Fe', 'Co', 'Ni', 'Cu', 'Zn', '#Ga', '#Ge', 'As', '#Se', 'Br',
'#Kr', '#Rb', 'Sr', '#Y', '#Zr', '#Nb', '#Mo', '#Tc', '#Ru', 'Rh', '#Pd',
'Ag', 'Cd', '#In', 'Sn', '#Sb', '#Te', 'I', '#Xe', '#Cs', 'Ba', '#La', '#Hf',
'#Ta', '#W', '#Re', '#Os', '#Ir', '#Pt', '#Au', 'Hg', '#Tl', 'Pb', '#Bi',
'#Po', '#At', '#Rn', '#Fr', '#Ra', '#Ac', '#Rf', '#Db', '#Sg', '#Bh', '#Hs',
'#Mt', '#Ds', '#Rg', '#Cn', '#Nh', '#Fl', '#Mc', '#Lv', '#Ts', '#Og']
'''
= [elem for elem in all_elements if not '#' in elem]
elements
print('elements = ', elements)
elements = ['N', 'O', 'F', 'Ne', 'Na', 'Mg', 'Al', 'Si', 'P', 'S', 'Cl', 'K', 'Ca', 'Ti', 'V', 'Cr', 'Mn', 'Fe', 'Co', 'Ni', 'Cu', 'Zn', 'As', 'Br', 'Sr', 'Rh', 'Ag', 'Cd', 'Sn', 'I', 'Ba', 'Hg', 'Pb']
; plot_patterns()
In the previous section, out of one million spectra, we have cherry picked 22 hotmax spectra and within each spectrum detected potentially significant peaks exceeding the Poisson noise level. We can now proceed to solve the peak pattern puzzle for each hotmax spectrum. In other words, for each spectrum explain the presence of each significant (numbered) peak. Can we attribute a given peak to a specific chemical element, the instrument or noise?
In other words, we can start to ‘explain away’ all peaks. It is highly instructive to walk through some interesting hotmax spectra and see which element patterns explain the peak patterns that we observe. To do so, import the plot_puzzle()
function.
from maxrf4u import plot_puzzle, plot_ptrn
= plot_puzzle('RP-T-1898-A-3689.datastack', 0, color_select=['Ca']) fig, ax_ptrns, ax_spectr
RP-T-1898-A-3689.datastack:
/
├── compton_peak_energy (1,) float64
├── hotmax_peak_idxs_list (22, 3) int64
├── hotmax_spectra (22, 4096) float32
├── hotmax_spots (22, 2) int64
├── imvis_extent (4,) int64
├── imvis_reg (1692, 1592, 4) float32
├── imvis_reg_highres (4920, 4629, 4) float32
├── maxrf_cube (1692, 1592, 4096) float32
├── maxrf_energies (4096,) float64
├── maxrf_maxspectrum (4096,) float32
├── maxrf_sumspectrum (4096,) float64
└── test_list (3, 3) int64
--------------------------------------------------------------------------- AssertionError Traceback (most recent call last) Cell In[7], line 1 ----> 1 fig, ax_ptrns, ax_spectr = plot_puzzle('RP-T-1898-A-3689.datastack', 0, color_select=['Ca']) File ~/Work/dev/maxrf4u/maxrf4u/peakpuzzle.py:250, in plot_puzzle(datastack_file, n, elements, color_select, footspace) 247 elements = eoi 249 elem_ptrns = get_patterns(elements=elements, color_select=color_select) --> 250 instrum_ptrn = get_instrument_pattern(datastack_file) 252 # prepare figure 253 n_ptrns = len(elements) + 1 File ~/Work/dev/maxrf4u/maxrf4u/peakpuzzle.py:306, in get_instrument_pattern(datastack_file) 303 keV1 = maxrf4u.RHODIUM_Ka 304 theta = maxrf4u.detector_angle(keV0, keV1) --> 306 sensor_peak_idx = ds.read('hotmax_pixels')[0, 2] 307 sensor_peak_keV = x_keVs[sensor_peak_idx] 310 anode_emission = mos.XFluo('Rh', tube_keV=30, min_prom=0.1) # only largest K peaks File ~/Work/dev/maxrf4u/maxrf4u/storage.py:324, in DataStack.read(self, datapath, latest, compute) 321 dataset = None 323 self.tree() --> 324 assert False, f'Dataset not found: {datapath}' 326 return dataset AssertionError: Dataset not found: hotmax_pixels
In the puzzle plot for hotmax spectrum #0 above, beside instrument peaks, all other peaks are rather small. I would say that only sub peak (4) can be explained as the \(K_{\alpha}\) emission of calcium.
'RP-T-1898-A-3689.datastack', 2, elements=['Pb', 'As', 'S'], color_select=['Pb', 'Ca', 'Fe', 'S']); plot_puzzle(
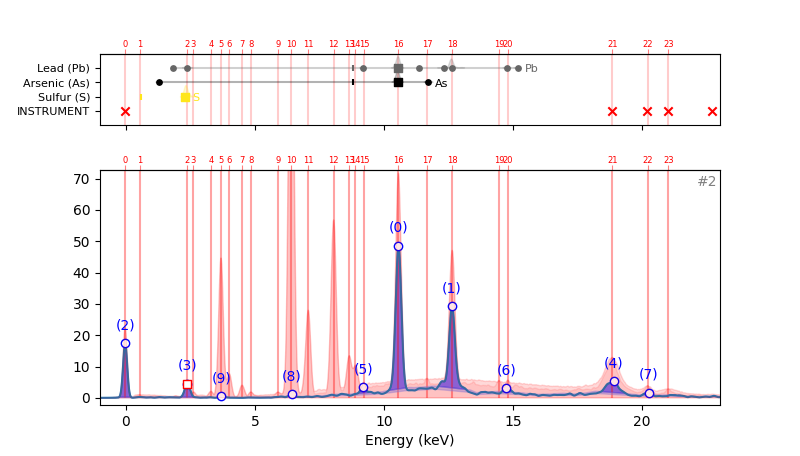
In hotmax spectrum #2 above, one can see that sub peaks [0], [1], [3] and [6] can all be explained by the emission of lead (Pb). The tiny peaks [8] and [9] are explained by respectively iron (Fe) and calcium (Ca).
'RP-T-1898-A-3689.datastack', 3, color_select=['Cl', 'Ca', 'Fe', 'Zn']); plot_puzzle(
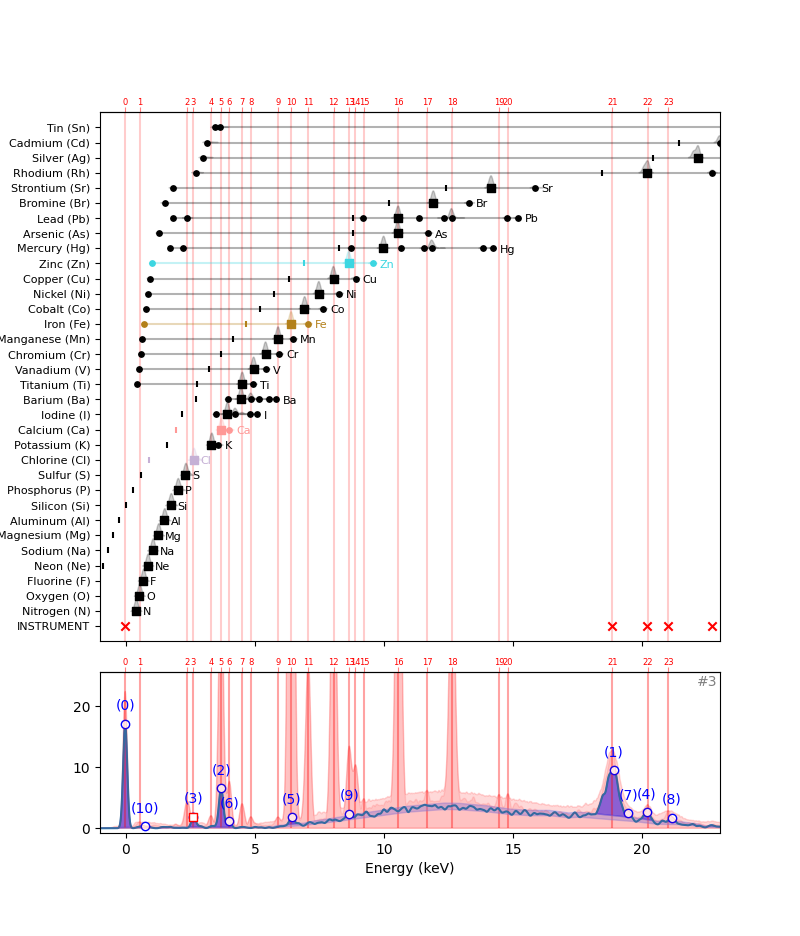
In hotmax spectrum #3 sub peak [3] interestingly indicates the presence of chlorine (Cl). Furthermore we find evidence for calcium (Ca), iron (Fe), and perhaps zinc (Zn).
= 0
n = plot_puzzle(hma, n)
ax0, ax1
# patterns
'Ca', -1, ax1); plot_ptrn(
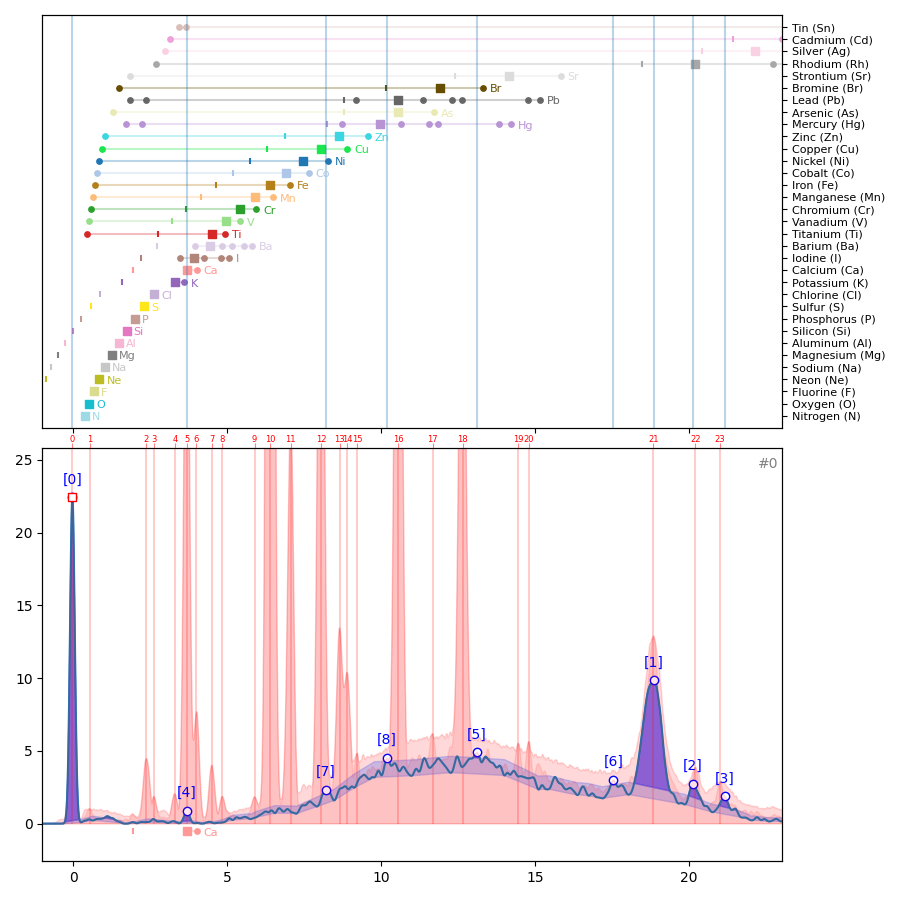
= 1
n = plot_puzzle(hma, n)
ax0, ax1
# patterns
'O', -1, ax1);
plot_ptrn('Ca', -1, ax1); plot_ptrn(
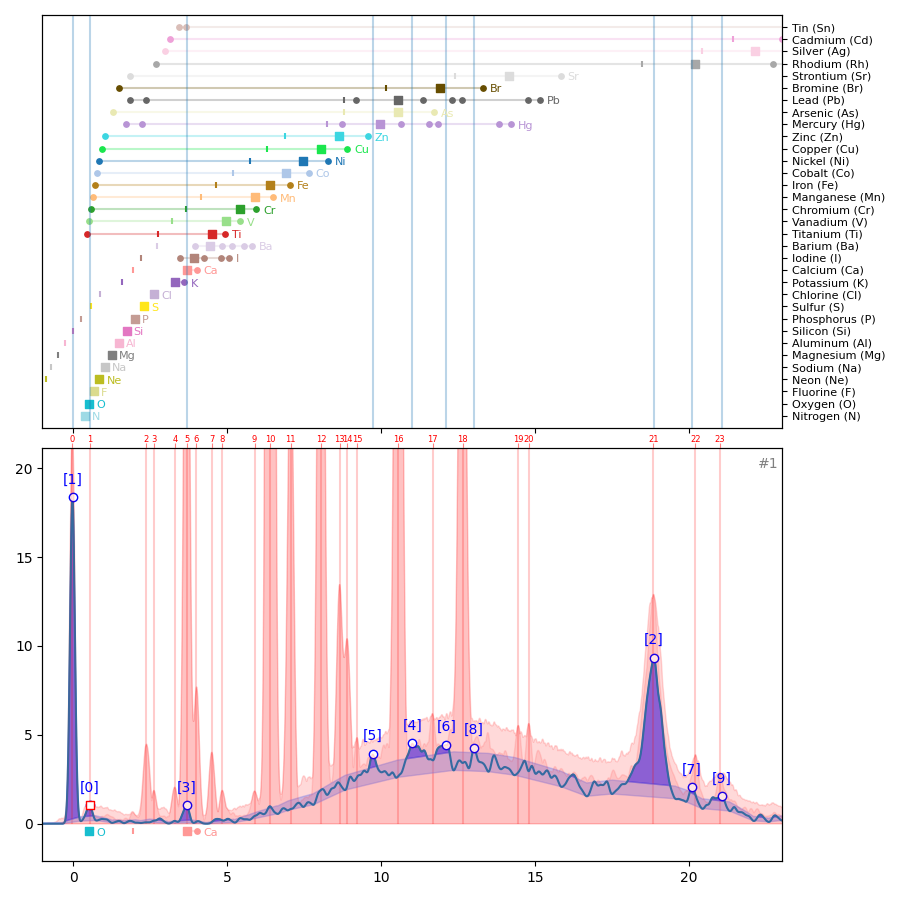
= 2
n = plot_puzzle(hma, n)
ax0, ax1
# patterns
'Pb', -1, ax1); plot_ptrn(
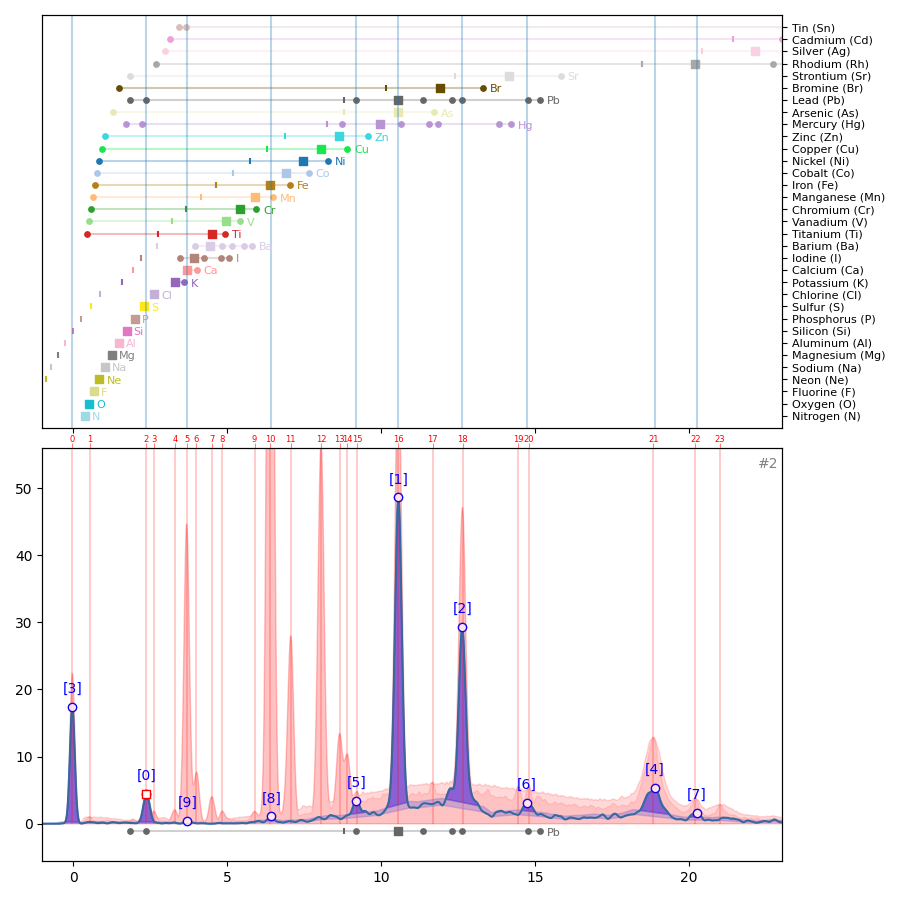
= 3
n = plot_puzzle(hma, n)
ax0, ax1
# patterns
'Cl', -1, ax1);
plot_ptrn('Ca', -1, ax1);
plot_ptrn('Fe', -2, ax1); plot_ptrn(
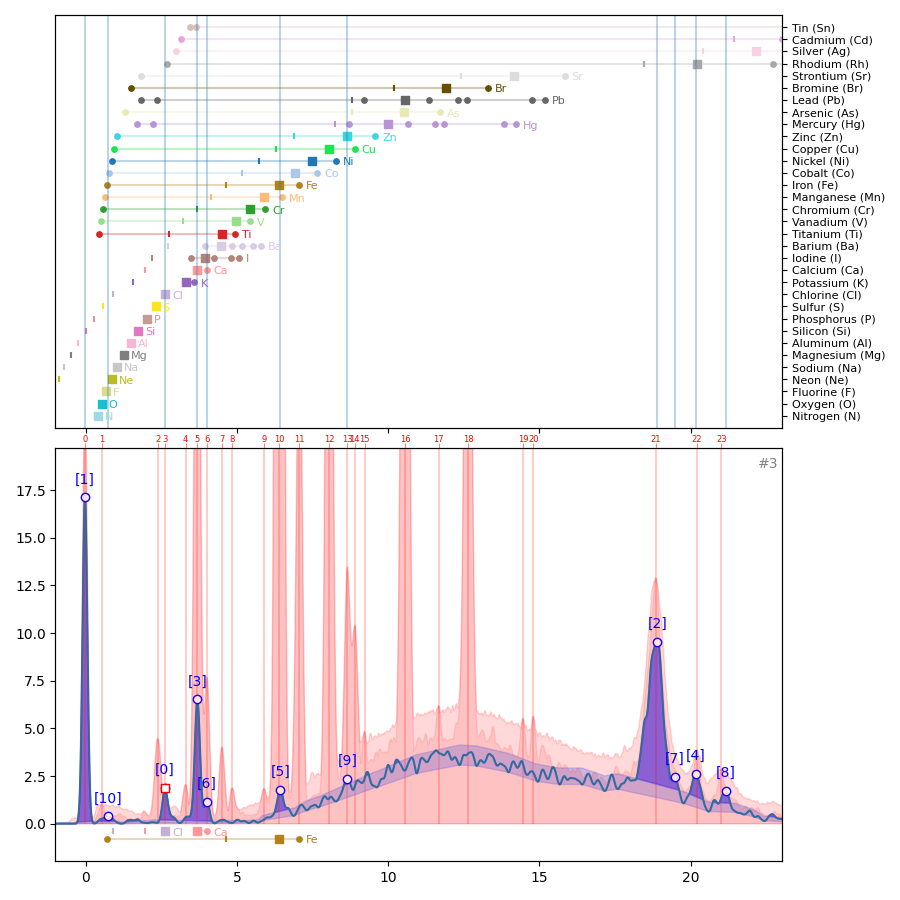
= 4
n = plot_puzzle(hma, n)
ax0, ax1
# patterns
'Fe', -3, ax1)
plot_ptrn('Ca', -1, ax1)
plot_ptrn('O', -1, ax1)
plot_ptrn('S', -1, ax1)
plot_ptrn('K', -2, ax1); plot_ptrn(
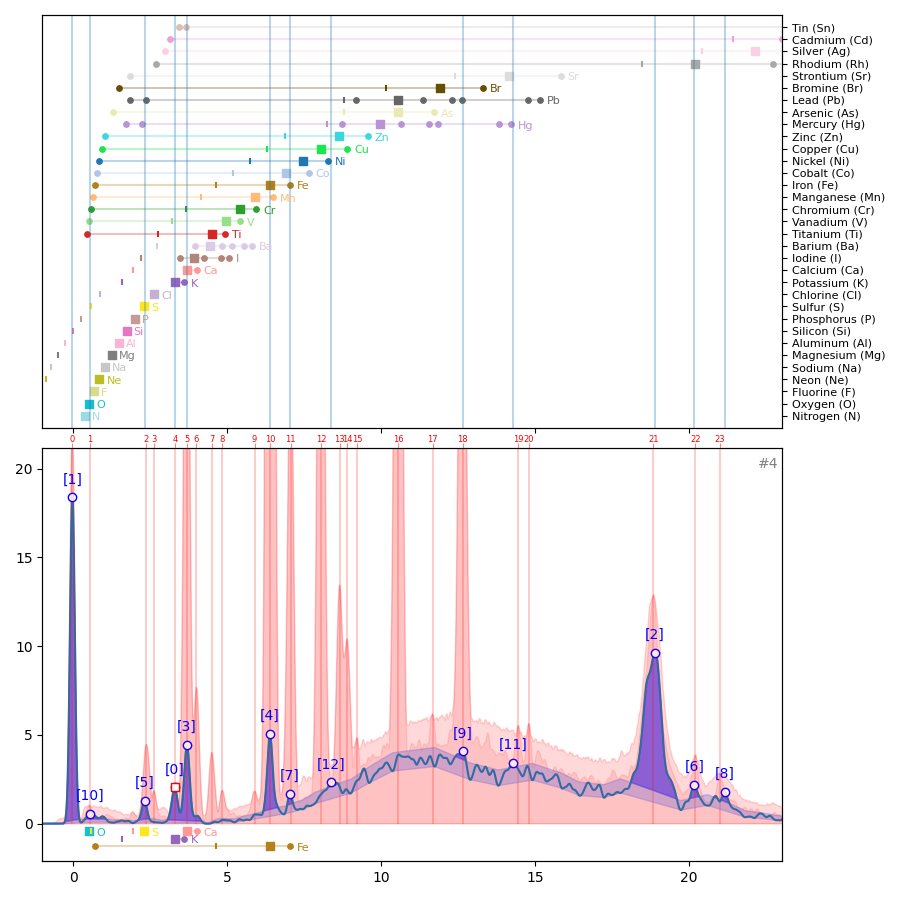
= 5
n = plot_puzzle(hma, n)
ax0, ax1
# patterns
'Ca', -1, ax1); plot_ptrn(
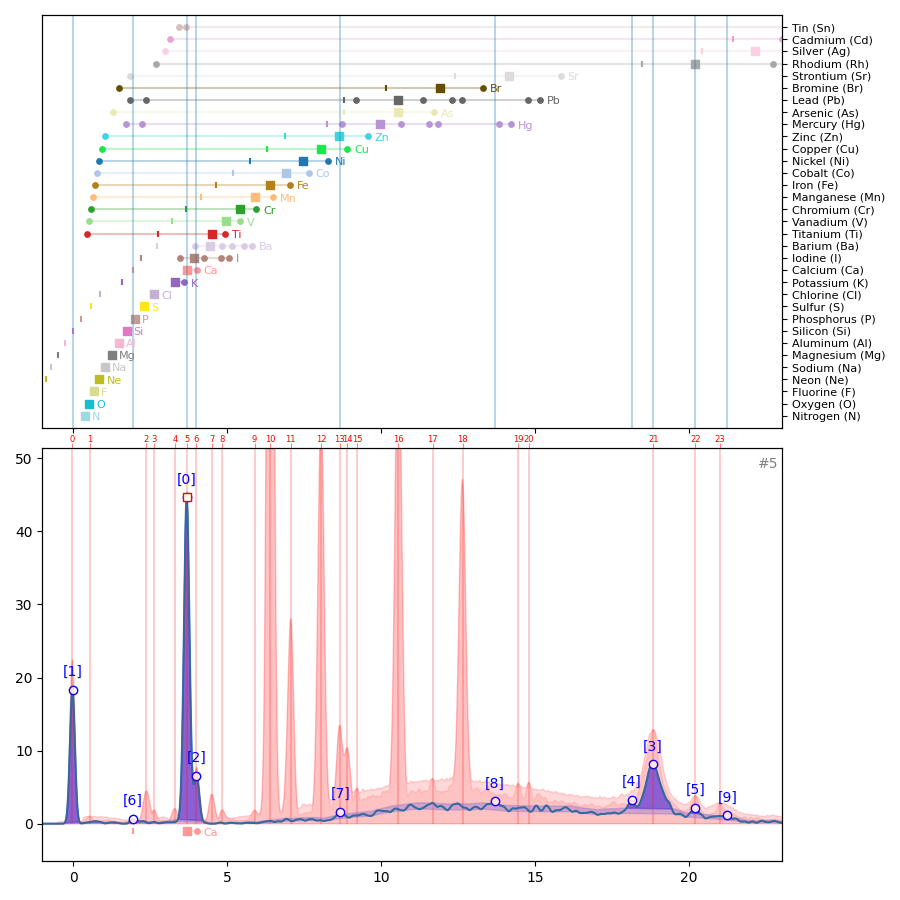
= 6
n = plot_puzzle(hma, n)
ax0, ax1
# patterns
'Ca', -1, ax1); plot_ptrn(
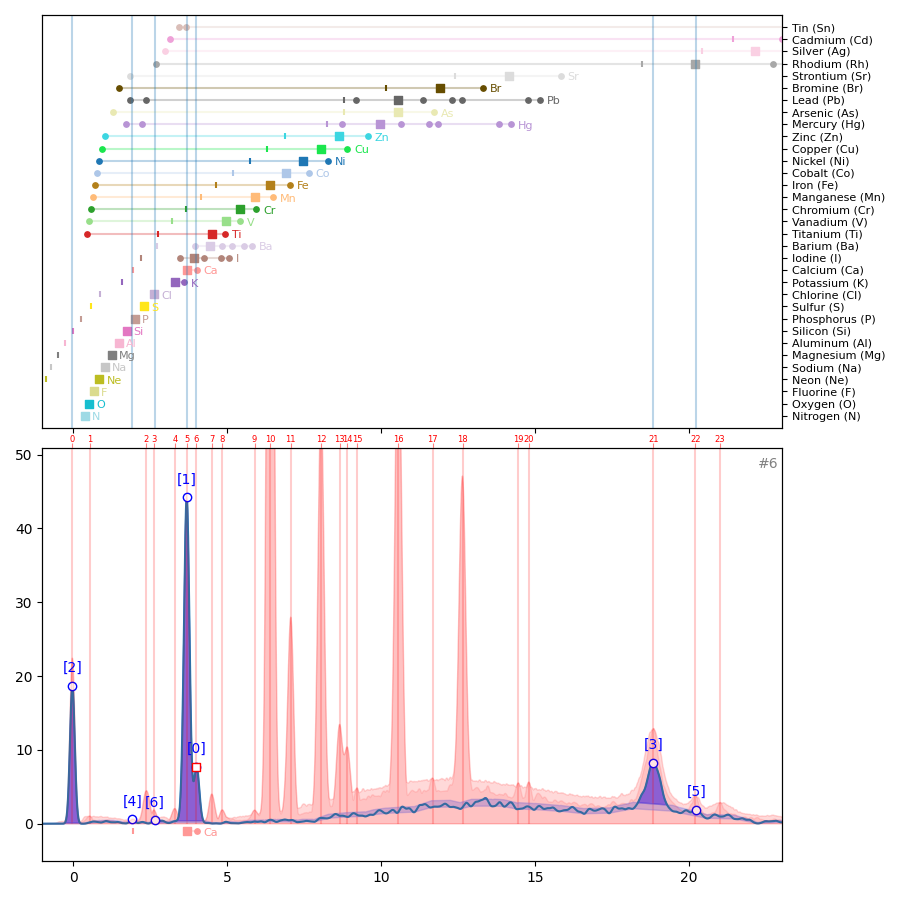
= 7
n = plot_puzzle(hma, n)
ax0, ax1
# patterns
'Fe', -1, ax1);
plot_ptrn('Ti', -2, ax1);
plot_ptrn('Ca', -3, ax1); plot_ptrn(
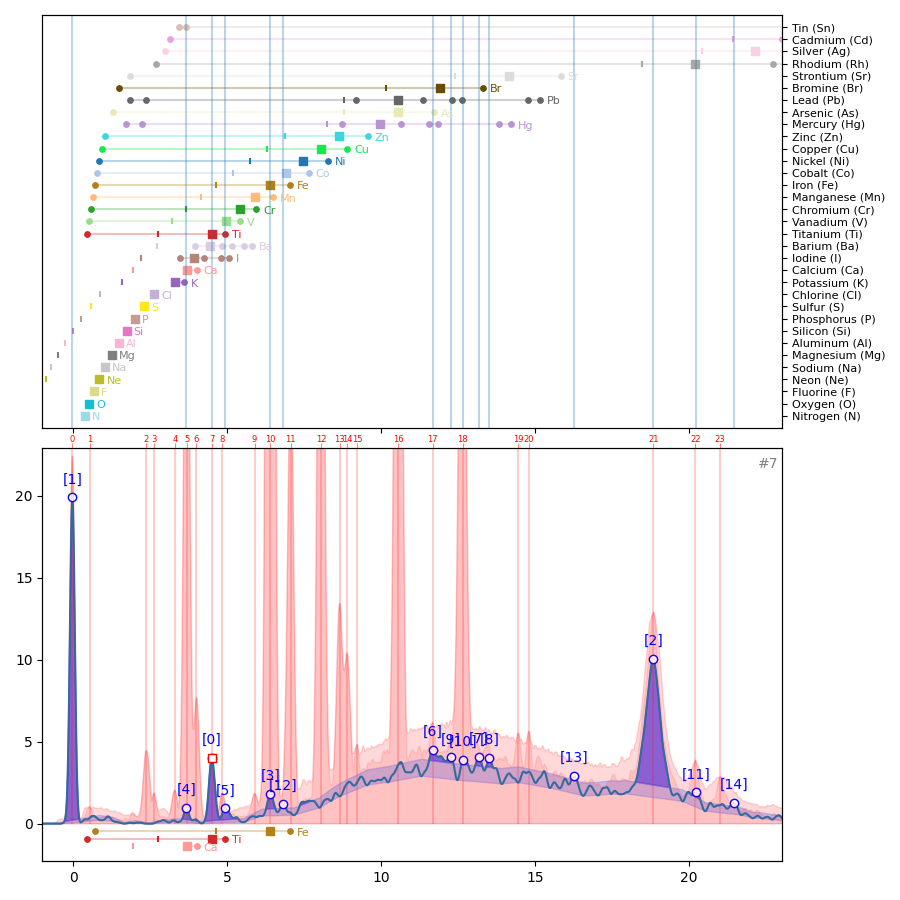
= 8
n = plot_puzzle(hma, n)
ax0, ax1
# patterns
'Fe', -3, ax1);
plot_ptrn('Ba', -1, ax1);
plot_ptrn('Ca', -2, ax1); plot_ptrn(
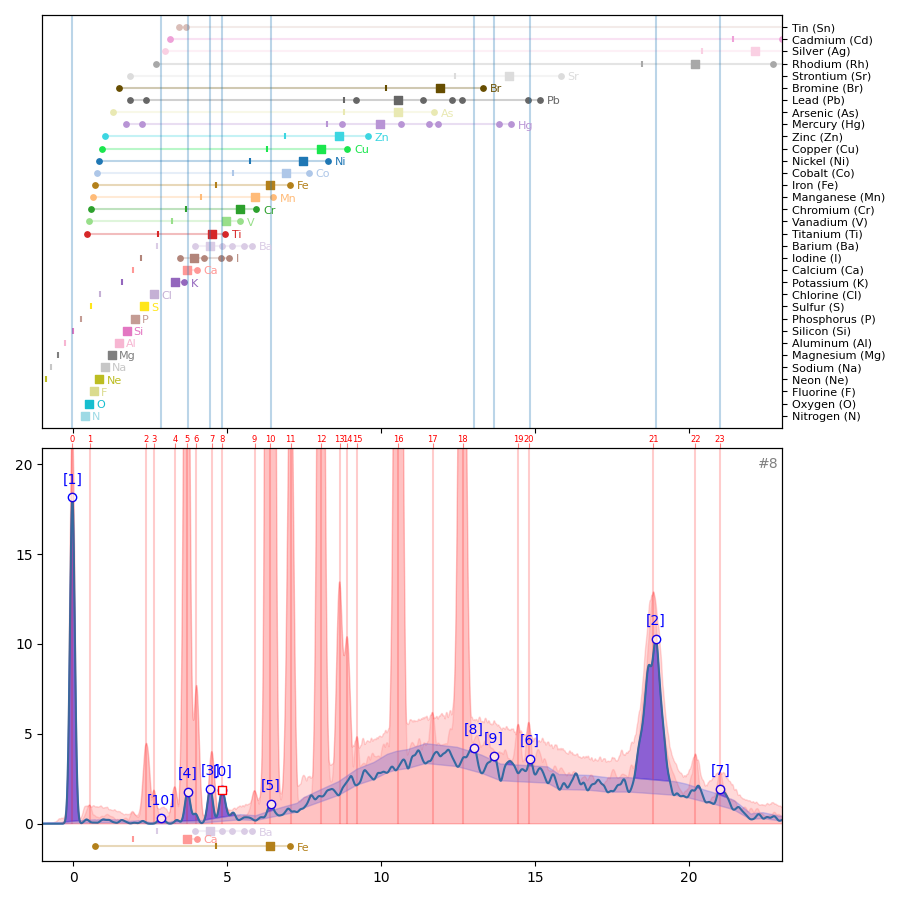
= 9
n = plot_puzzle(hma, n)
ax0, ax1
# patterns
'Mn', -1, ax1);
plot_ptrn('Ca', -2, ax1);
plot_ptrn('Fe', -3, ax1); plot_ptrn(
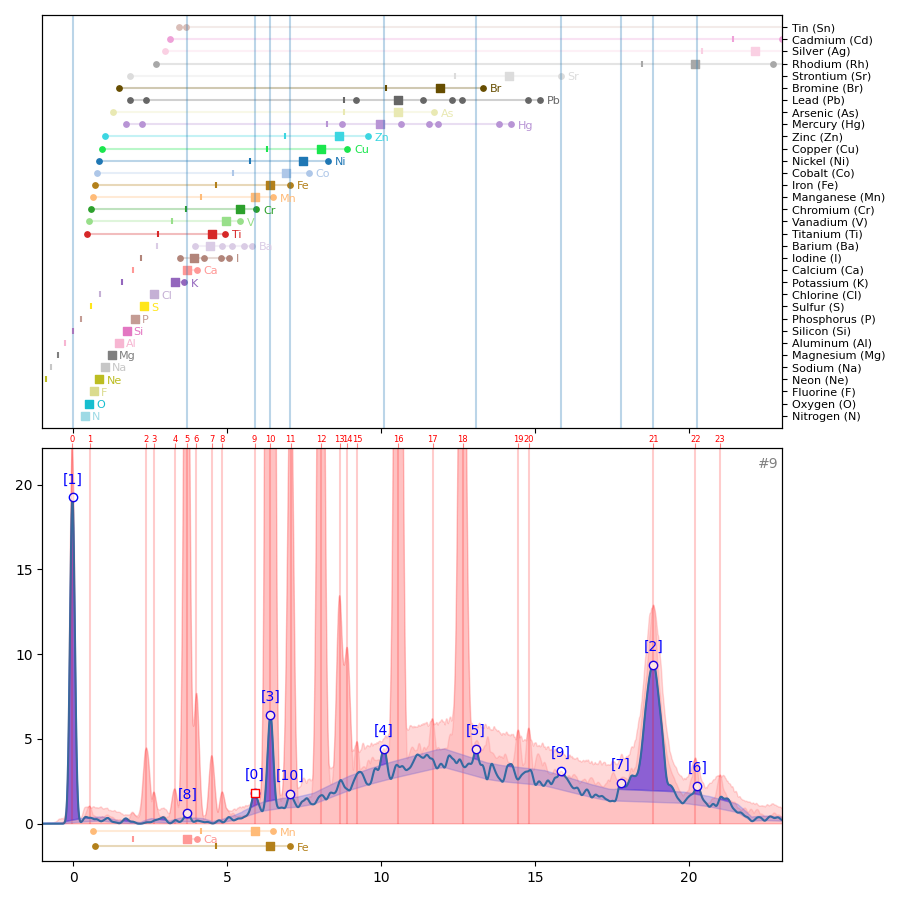
= 10
n = plot_puzzle(hma, n)
ax0, ax1
# patterns
'Fe', -1, ax1);
plot_ptrn('Ca', -2, ax1); plot_ptrn(
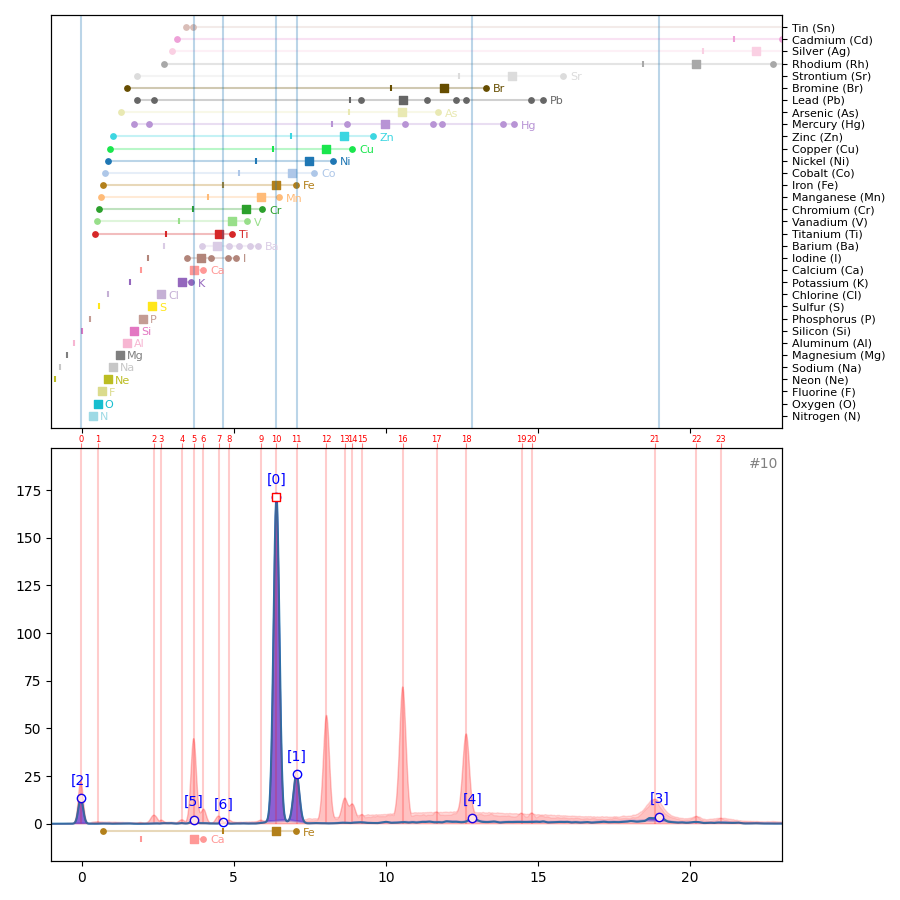
The tiny peak [6] in hotmax spectrum #10 is clearly the escape peak for Fe located at 6.40 keV minus 1.74 keV.
= 11
n = plot_puzzle(hma, n)
ax0, ax1
# patterns
'Fe', -1, ax1);
plot_ptrn('Ca', -2, ax1); plot_ptrn(
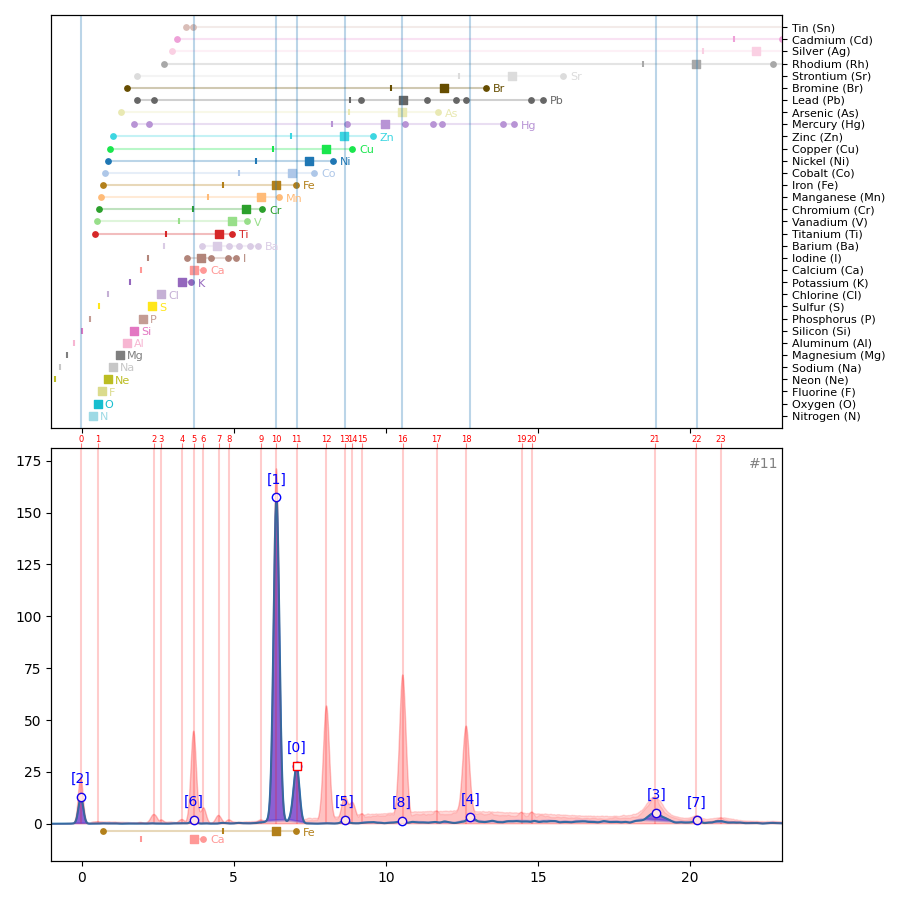
= 12
n = plot_puzzle(hma, n)
ax0, ax1
# patterns
'Cu', -1, ax1);
plot_ptrn('Zn', -2, ax1);
plot_ptrn('Ca', -3, ax1); plot_ptrn(
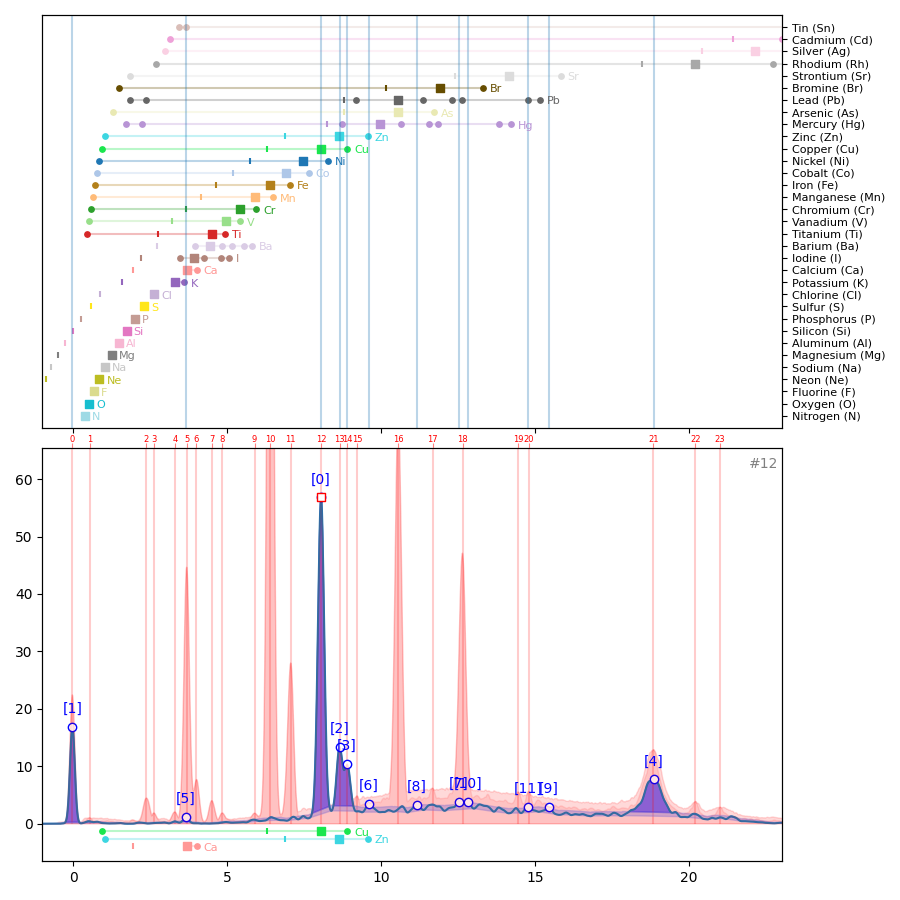
= 13
n = plot_puzzle(hma, n)
ax0, ax1
# patterns
'Cu', -1, ax1);
plot_ptrn('Zn', -2, ax1);
plot_ptrn('Ca', -3, ax1); plot_ptrn(
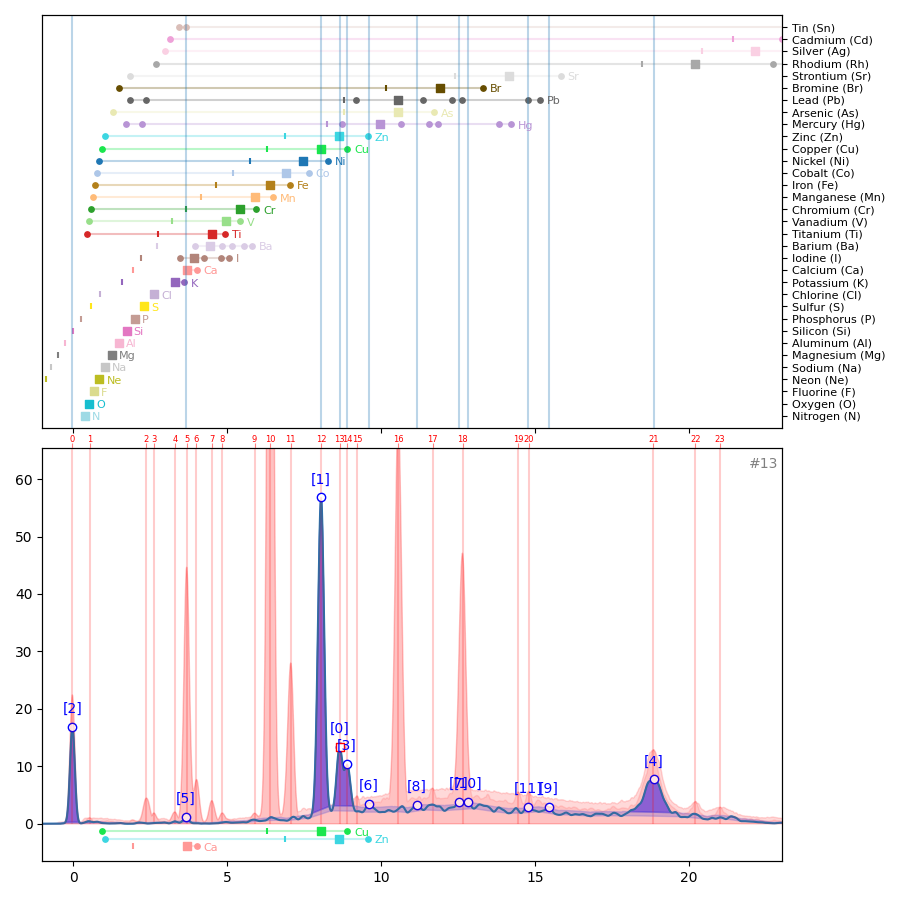
= 14
n = plot_puzzle(hma, n)
ax0, ax1
# patterns
'Cu', -1, ax1);
plot_ptrn('Zn', -2, ax1);
plot_ptrn('Ca', -3, ax1); plot_ptrn(
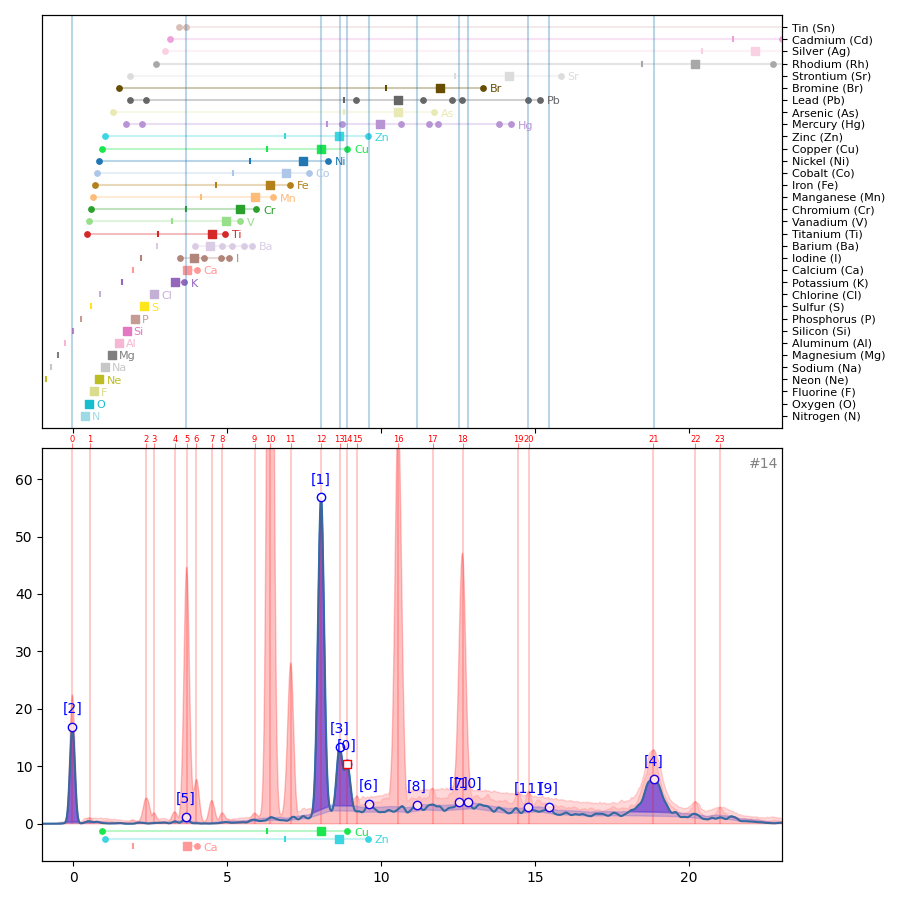
= 15
n = plot_puzzle(hma, n)
ax0, ax1
# patterns
'Pb', -1, ax1); plot_ptrn(
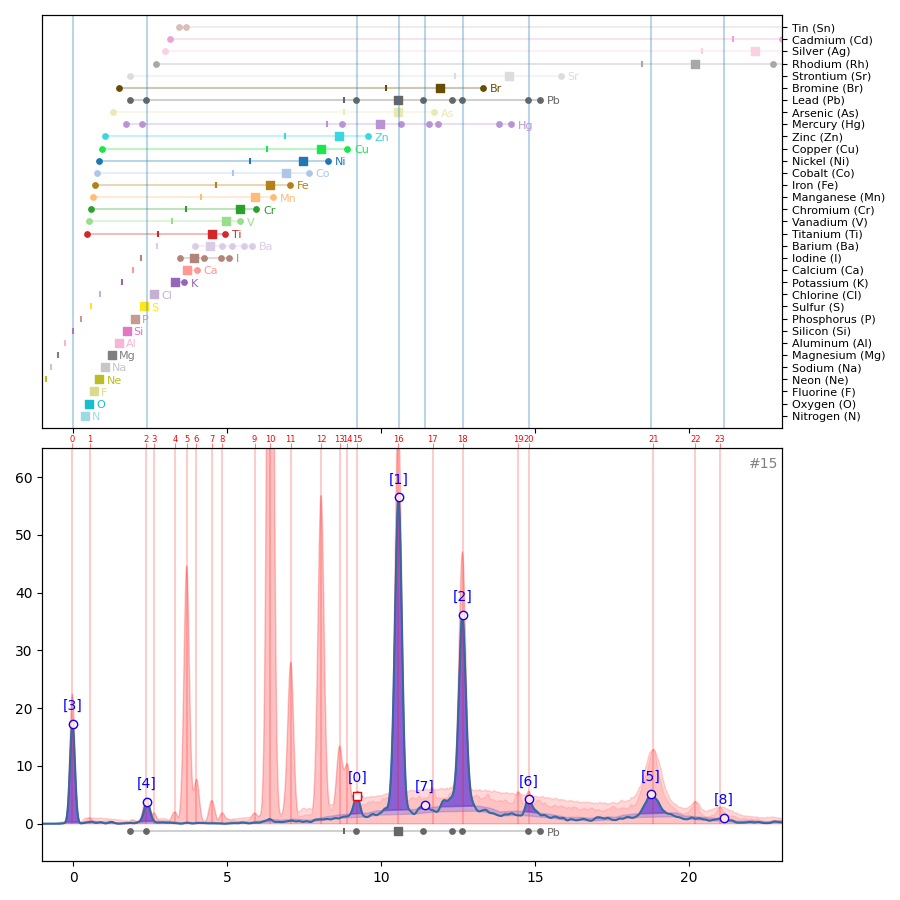
= 16
n = plot_puzzle(hma, n)
ax0, ax1
# patterns
'Pb', -1, ax1); plot_ptrn(
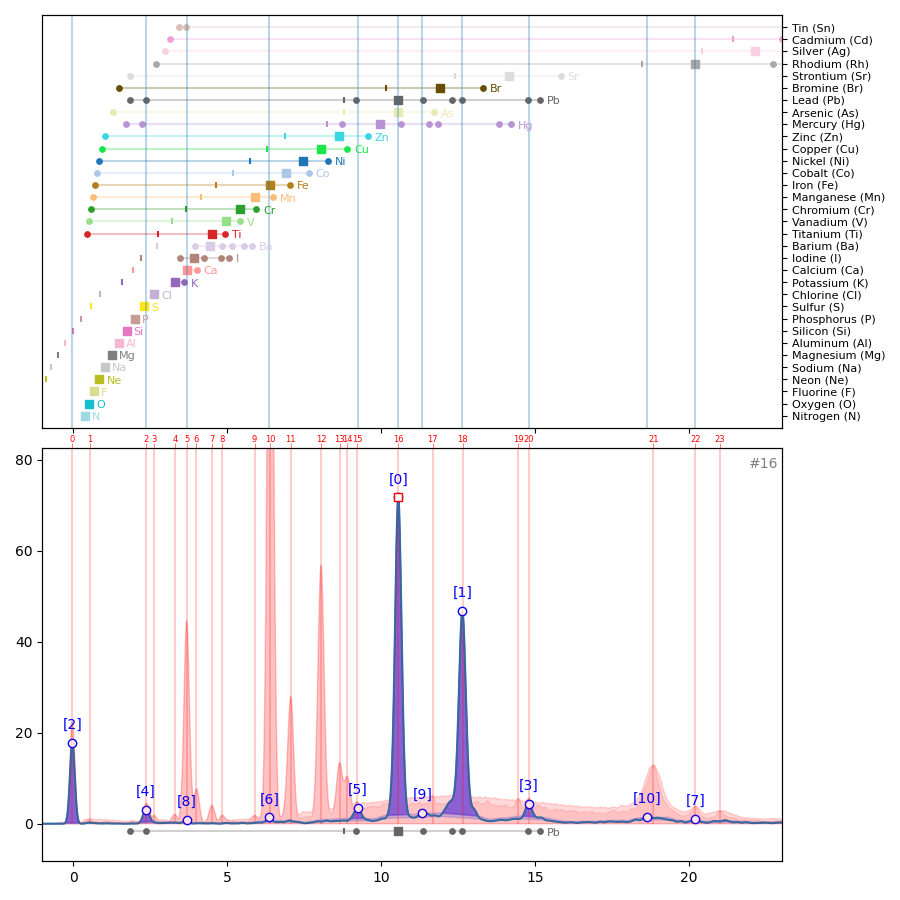
= 17
n = plot_puzzle(hma, n)
ax0, ax1
# patterns
'Ca', -1, ax1); plot_ptrn(
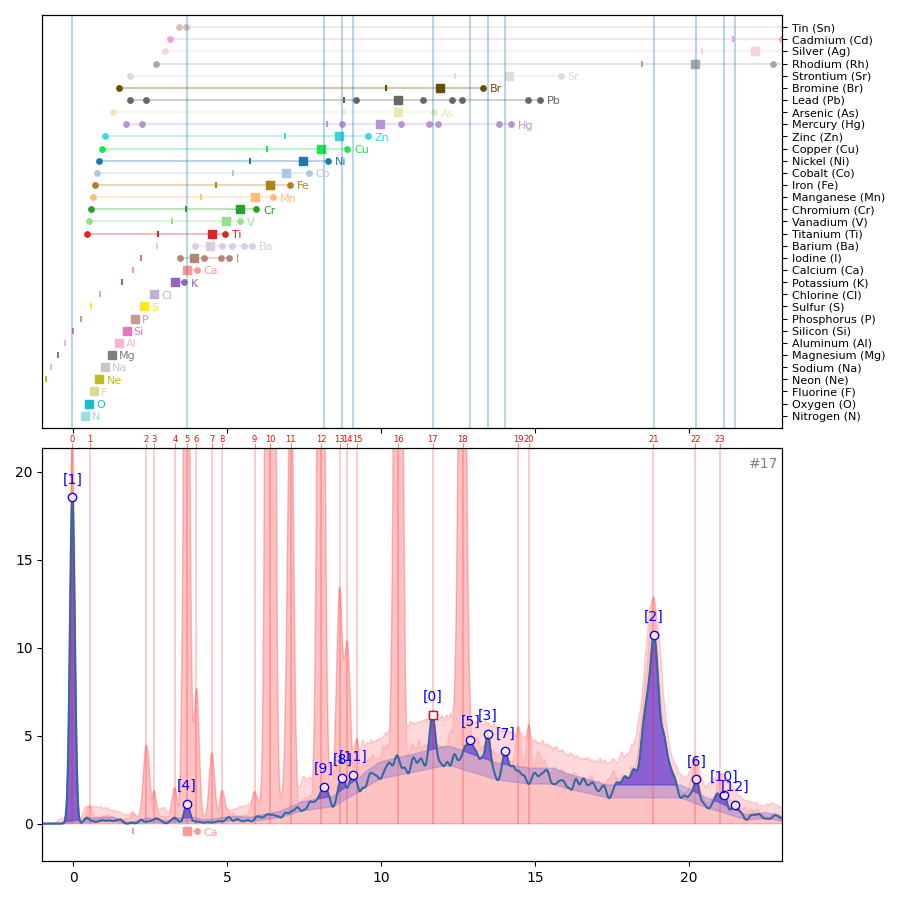
= 18
n = plot_puzzle(hma, n)
ax0, ax1
# patterns
'Pb', -1, ax1); plot_ptrn(
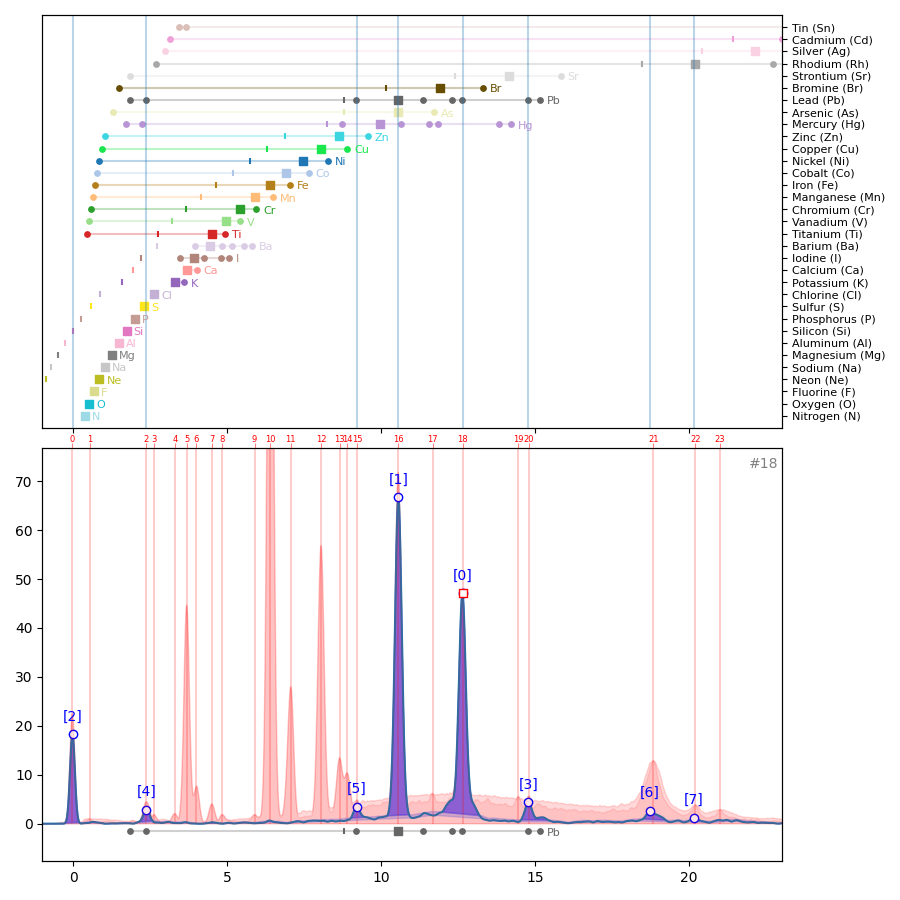
= 19
n = plot_puzzle(hma, n)
ax0, ax1
# patterns
'Ca', -1, ax1); plot_ptrn(
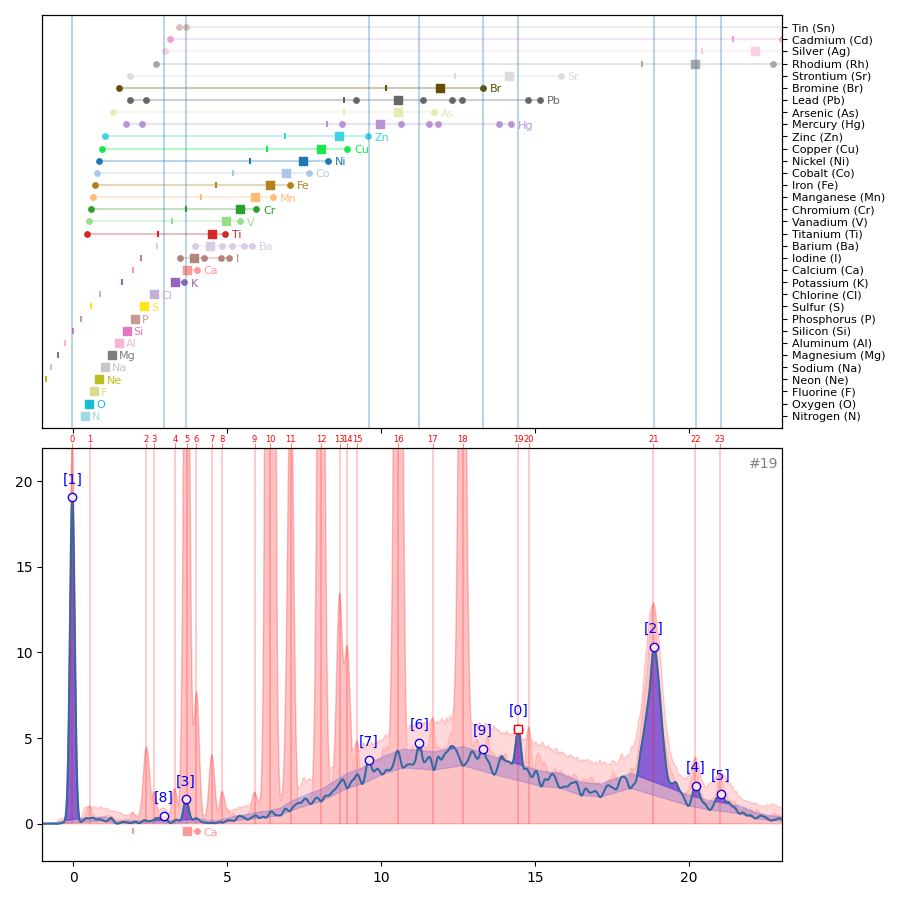
= 20
n = plot_puzzle(hma, n)
ax0, ax1
# patterns
'Pb', -1, ax1); plot_ptrn(
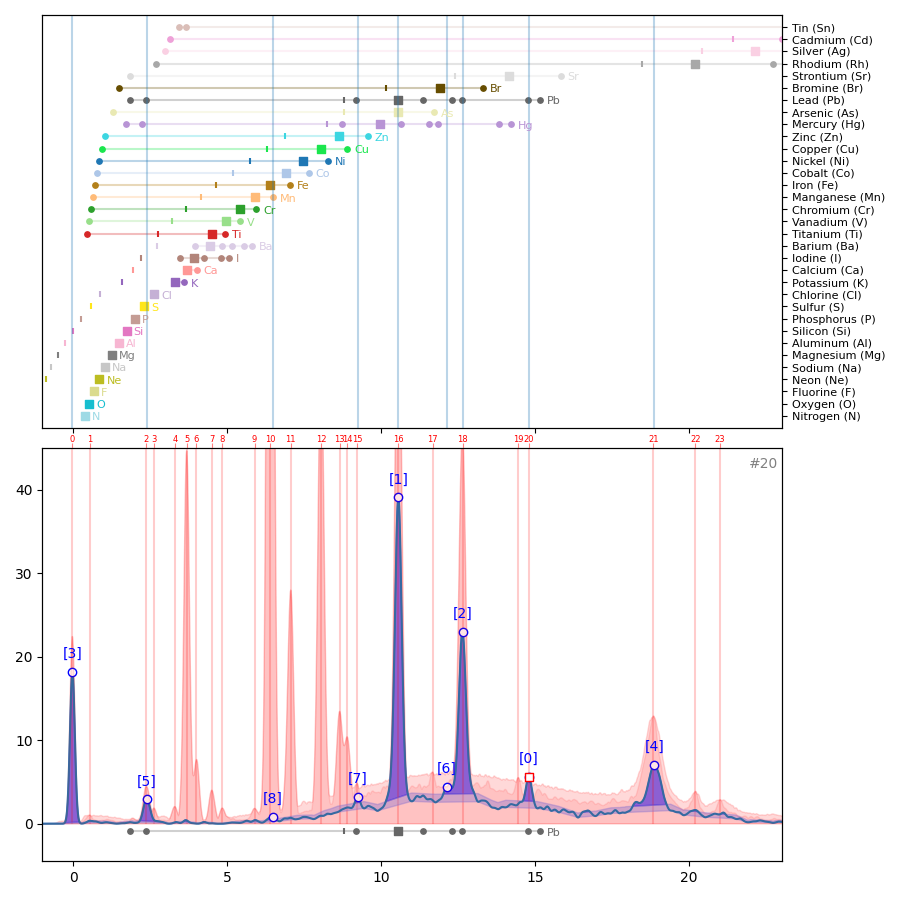
Ok, that is it. Let’s try to summarize what we have learned…
Summary
Code
import matplotlib.pyplot as plt
from maxrf4u import plot_patterns, get_patterns, DataStack
from maxrf4u.peakmaps import _add_hotlines_ticklabels
Code
= DataStack('RP-T-1898-A-3689.datastack')
ds
= ds.read('maxrf_energies')
x_keVs = ds.read('maxrf_maxspectrum')
y_max = ds.read('maxrf_sumspectrum')
y_sum = ds.read('hotmax_spectra')
hotmax_spectra
= ['S', 'Ca', 'K', 'Cl', 'Fe', 'Mn', 'Cu', 'Zn', 'Pb', 'Ti', 'Ba']
elements
#ptrn_list = get_patterns(['S', 'Ca', 'K', 'Cl', 'Fe', 'Mn', 'Cu', 'Zn', 'Pb', 'Ti', 'Ba'])
= plt.subplots(nrows=2, sharex=True, figsize=[7, 5])
fig, [ax, ax1]
=ax)
plot_patterns(elements, ax
'RP-T-1898-A-3689.datastack', ax, clip_vline=False)
_add_hotlines_ticklabels(
#plot_cube_slices('RP-T-1898-A-3689.datastack', ax=ax1);
='r', label='max spectrum')
ax1.plot(x_keVs, y_max, color='r', alpha=0.3)
ax1.fill_between(x_keVs, y_max, color
for y_hot in hotmax_spectra:
=[0.2, 0.1, 0.8], linewidth=0.5)
ax1.plot(x_keVs, y_hot, color#ax1.plot(x_keVs, y_sum, color=[0.3, 1, 0.3], label='sum spectrum')
'RP-T-1898-A-3689.datastack', ax1)
_add_hotlines_ticklabels(
-1, 23])
ax1.set_xlim([-5, 100])
ax1.set_ylim([;
ax1.legend()
'Peak pattern summary')
ax.set_title( plt.tight_layout()
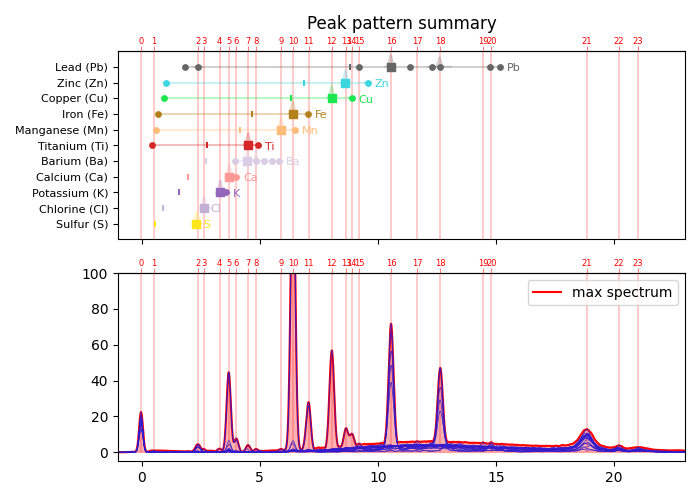
Altogether the spectral data indicates that 11 chemical elements are present in the Susanna drawing: sulfur (S), chlorine (Cl), potassium (K), calcium (Ca), barium (Ba), titanium (Ti), manganese (Mn), iron (Fe), copper (Cu), zinc (Zn) and lead (Pb).
In the next section we will look into the spatial distribution of these elements…
FUNCTIONS
add_hotlines
add_hotlines (datastack_file, ax, vlines=True, clip_vline=True)
*Utility function. Adds hotlines and tick labels to plot ax
.
Instrument peaks are colored black.*
get_instrument_pattern
get_instrument_pattern (datastack_file)
*Generate instrument peak pattern.
Pattern dictionary contains strongest Rhodium anode emission peaks,
their corresponding Compton shifted peaks, and a sensor peak
Returns: instrument_pattern_dict*
plot_puzzle
plot_puzzle (datastack_file, n, elements=None, color_select=None, footspace=0.2)
*Plot peak pattern puzzle for hotmax spectrum n
with emission patterns.
If elements
is specified, only plot patterns for those elements.*
plot_patterns
plot_patterns (elements=None, instr_ptrn_from_datastack_file=None, color_select=None, ax=None)
*Wrapper function to plot overview of element
and instrument patterns in axes ax
.
Returns: ax
*
plot_ptrn
plot_ptrn (elem, y, ax, color_select=None)
Low level plot element pattern at level y
in axes ax
.
colorize
colorize (elem)
Pick fixed color from nice color map for elements of interest.
get_patterns
get_patterns (elements=None, tube_keV=30, color_select=None)
*Returns pattern dict list for elements
list, sorted according to alpha peak energy.
By default all elements are colored with a standard color. To colorize only specific elements, provide a list of elements color_select
.*